What is the Use of Artificial Intelligence in Autonomous Vehicles
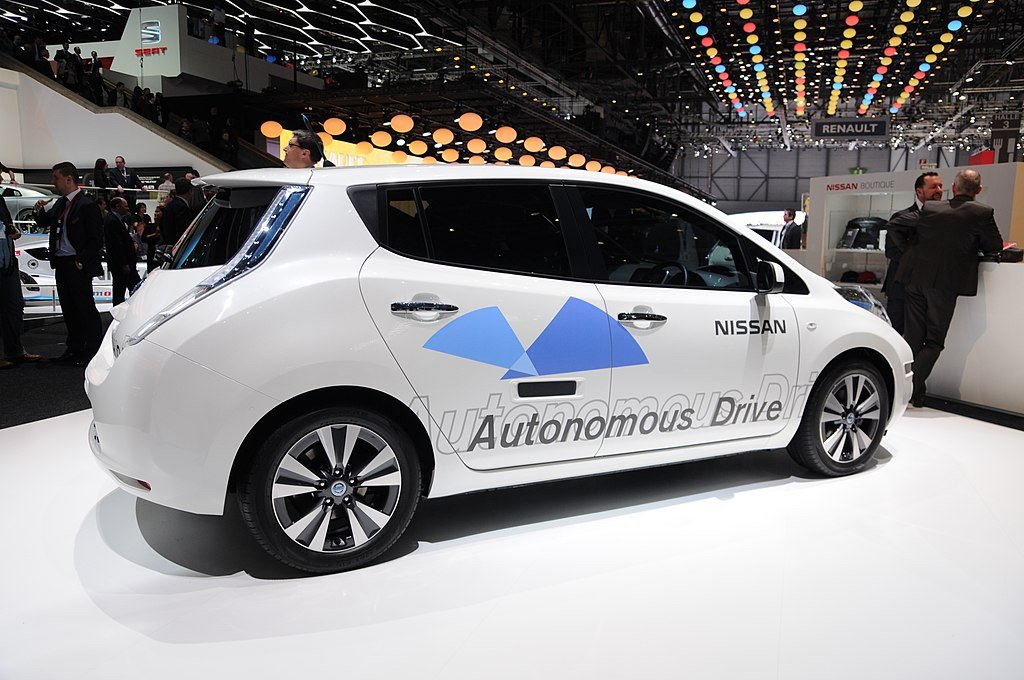
The Use of Artificial Intelligence in Autonomous Vehicles
The development of autonomous vehicles (AVs), often referred to as self-driving cars, is one of the most transformative innovations in modern transportation. These vehicles are equipped with AI technologies that allow them to operate without human intervention. Artificial intelligence (AI) plays a pivotal role in enabling AVs to navigate, perceive their environment, make decisions, and continuously improve their functionality. The integration of AI into autonomous vehicles has far-reaching implications, from improving road safety to transforming the way we commute and reshaping industries like logistics and mobility.
In this article, we will explore how AI is used in autonomous vehicles, the types of AI technologies involved, and some of the sample apps or products that leverage AI in autonomous vehicles.
What is Artificial Intelligence in Autonomous Vehicles?
Artificial intelligence in autonomous vehicles refers to the systems, algorithms, and technologies that allow a vehicle to operate autonomously by perceiving the world around it, interpreting sensor data, making decisions, and controlling the vehicle’s movement, all without human input. This technology uses a combination of sensors, cameras, radar, machine learning, and neural networks to mimic human driving capabilities and decision-making processes.
There are five levels of vehicle automation defined by the SAE (Society of Automotive Engineers) standards:
- Level 0: No automation (manual driving)
- Level 1: Driver assistance (e.g., cruise control, lane assist)
- Level 2: Partial automation (e.g., Tesla’s Autopilot)
- Level 3: Conditional automation (e.g., Audi’s Traffic Jam Pilot)
- Level 4: High automation (e.g., Waymo’s fully autonomous vehicles in specific locations)
- Level 5: Full automation (no human intervention required)
AI primarily supports levels 3 to 5, where vehicles can drive independently of human intervention in most situations. The underlying AI technologies used in autonomous vehicles include deep learning, computer vision, reinforcement learning, sensor fusion, and more.
Key AI Technologies in Autonomous Vehicles
AI in autonomous vehicles is composed of several core technologies that work together to ensure safe and efficient operation. Some of the key AI technologies are:
1. Computer Vision
Computer vision is the field of AI that enables machines to interpret and understand visual information from the world. In autonomous vehicles, computer vision uses cameras and sensors to detect and recognize objects such as other vehicles, pedestrians, traffic signs, road markings, traffic lights, and obstacles. This technology is crucial for perception, allowing the vehicle to understand its surroundings and make informed decisions.
Computer vision algorithms typically use convolutional neural networks (CNNs) to analyze the images captured by cameras and extract useful features for decision-making.
2. Lidar and Radar
Lidar (Light Detection and Ranging) and radar (Radio Detection and Ranging) are sensor technologies that provide critical data for autonomous vehicles. Lidar uses laser pulses to measure distances and generate 3D maps of the environment, while radar uses radio waves to detect objects and their velocities. These sensors help vehicles “see” beyond the limitations of cameras, especially in low visibility conditions such as fog, rain, or at night.
AI algorithms process the data from these sensors to build a detailed representation of the environment and detect objects in real-time.
3. Machine Learning
Machine learning is a subset of AI that enables machines to learn from data and improve over time without being explicitly programmed. In autonomous vehicles, machine learning algorithms analyze vast amounts of data from sensors, cameras, and lidar to recognize patterns and make predictions. These algorithms are continuously trained using real-world driving data to improve their performance.
Reinforcement learning, a type of machine learning, plays a vital role in decision-making by enabling the vehicle to learn optimal actions through trial and error. This is especially important for tasks such as path planning, where the vehicle needs to learn how to navigate complex road environments efficiently.
4. Sensor Fusion
Sensor fusion refers to the process of combining data from multiple sensors (such as cameras, radar, lidar, GPS, and ultrasonic sensors) to create a more accurate and comprehensive understanding of the vehicle’s surroundings. AI plays a critical role in sensor fusion by merging raw data into a unified perception model.
By integrating data from different sources, sensor fusion helps autonomous vehicles mitigate the limitations of individual sensors and improves the reliability of object detection and classification.
5. Natural Language Processing (NLP)
Natural language processing (NLP) enables autonomous vehicles to interpret and respond to voice commands or interact with passengers. NLP can be used for voice-activated systems to control the vehicle’s features, such as adjusting the air conditioning, changing routes, or responding to queries. This technology enhances the user experience and provides more intuitive interaction with the vehicle.
6. Path Planning and Decision Making
Path planning involves determining the safest and most efficient route for the vehicle to follow while avoiding obstacles and responding to dynamic changes in the environment. AI algorithms in autonomous vehicles use a variety of methods, such as the A* algorithm and Rapidly-exploring Random Trees (RRT), to plan and execute driving maneuvers.
Decision-making algorithms, often based on probabilistic models, assess the current state of the environment and select the best course of action, whether that be slowing down, changing lanes, or stopping. These algorithms must be highly reliable to ensure safety in complex driving scenarios.
Applications of AI in Autonomous Vehicles
Autonomous vehicles rely on AI in various ways to enhance their functionality, improve safety, and provide a better driving experience. Here are some common applications of AI in autonomous vehicles:
1. Autonomous Driving Systems
Autonomous driving systems use AI to control the vehicle’s acceleration, steering, and braking based on inputs from the environment. These systems continuously process data from sensors and cameras to make real-time decisions about vehicle movements, ensuring smooth and safe driving without human intervention.
For example, Tesla’s Autopilot system uses machine learning algorithms to provide semi-autonomous driving capabilities such as lane centering, adaptive cruise control, and automatic lane changes.
2. Safety Features
AI-powered safety features such as collision avoidance, emergency braking, and lane-keeping assist have significantly enhanced road safety. By constantly monitoring the environment and making split-second decisions, AI can prevent accidents and reduce the severity of collisions.
Some AVs are equipped with automatic emergency braking (AEB) systems that use AI to detect potential collisions and automatically apply the brakes to prevent or mitigate the impact.
3. Traffic Management
AI can optimize traffic flow by coordinating autonomous vehicles to move in concert with one another. Vehicles can communicate with each other using Vehicle-to-Vehicle (V2V) communication to share information about traffic conditions, obstacles, and road hazards. This allows autonomous vehicles to adjust their speed and trajectory for smoother travel and reduced congestion.
Additionally, AI can be used for smart traffic lights that adapt to the flow of traffic, reducing waiting times and improving overall road efficiency.
4. Driver Monitoring and Assistance
AI is also used in driver assistance systems that monitor the driver’s behavior and provide warnings if necessary. For instance, if a driver is not paying attention or showing signs of drowsiness, the system can alert them to take action or even take control of the vehicle in certain situations.
5. Fleet Management and Logistics
AI is also employed in autonomous vehicle fleets used for logistics and delivery services. AI systems help manage routes, track vehicles in real-time, optimize delivery times, and ensure efficient use of resources. Companies like Waymo and Cruise are testing autonomous vehicles for transporting goods, while others like Uber and Amazon are experimenting with autonomous delivery vans.
Sample Apps and Products Using AI in Autonomous Vehicles
- Waymo (by Alphabet) Waymo is a leader in autonomous vehicle technology and has developed one of the most advanced self-driving platforms. Waymo’s AI-powered autonomous vehicles use a combination of lidar, radar, and cameras to navigate urban environments, identify objects, and make safe decisions in complex traffic situations. Waymo’s self-driving taxis are operational in select cities, providing ride-hailing services without human drivers.
- Tesla Autopilot Tesla’s Autopilot is one of the most well-known semi-autonomous driving systems on the market. It uses AI algorithms and deep learning to assist with lane-keeping, adaptive cruise control, and even automatic lane changes. Tesla’s vehicles also feature a Full Self-Driving (FSD) option, which, when fully activated, enables hands-free driving on highways and city streets.
- Cruise (acquired by General Motors) Cruise is a subsidiary of General Motors that focuses on developing autonomous vehicles. Cruise’s AI-powered self-driving cars are designed to navigate complex urban environments. The company has already begun testing its autonomous vehicles in San Francisco and aims to launch a fully autonomous ride-hailing service.
- Aurora Innovation Aurora Innovation is a leader in autonomous driving technology, focused on developing self-driving systems for freight transportation and passenger vehicles. Aurora’s AI technology uses sensor data and machine learning to enable safe navigation in challenging conditions such as night driving, construction zones, and inclement weather.
- Mobileye (acquired by Intel) Mobileye, now part of Intel, provides computer vision and machine learning technologies for autonomous vehicles. The company’s EyeQ platform is used in advanced driver-assistance systems (ADAS) and supports autonomous driving in a variety of vehicles. Mobileye’s AI technology enables cars to detect pedestrians, cyclists, and other vehicles, improving safety and enabling autonomous navigation.
Artificial intelligence is revolutionizing the automotive industry, particularly in the realm of autonomous vehicles. With technologies such as computer vision, machine learning, sensor fusion, and path planning, AVs are becoming increasingly capable of navigating complex environments and making real-time decisions. AI is driving innovation in safety, efficiency, and user experience, and is expected to play a crucial role in the widespread adoption of fully autonomous vehicles.
As companies like Waymo, Tesla, Cruise, and Mobileye continue to develop and refine their AI-powered self-driving systems, the future of transportation promises to be safer, more efficient, and more accessible. However, challenges remain, including regulatory hurdles, public acceptance, and the need for further advancements in AI to ensure the safe operation of autonomous vehicles in all conditions.
With continued research, collaboration, and testing, AI has the potential to reshape not just transportation but entire industries, from logistics and mobility to urban planning and infrastructure development. As we move toward a future with more autonomous vehicles, the role of AI in making these vehicles smarter, safer, and more efficient will only continue to grow. image/wikipedia